AI4Life joins FAIRsharing
by Beatriz Serrano-Solano
AI4Life has officially joined the FAIRsharing community. By registering our records on FAIRsharing, we are reaffirming our commitment to the FAIR principles, which are crucial for the sustainable management and sharing of data and AI models.
AI4Life’s contributions to FAIRsharing include a set of guidelines and resources designed to operationalize the FAIR principles within the context of AI and bioimaging. Some of the key resources and guidelines now available on FAIRsharing include:
BioImage Model Zoo Model Specification
A standard format for documenting and disseminating AI models, ensuring cross-compatibility with bioimaging tools and easy integration into research workflows. Explore the record here.
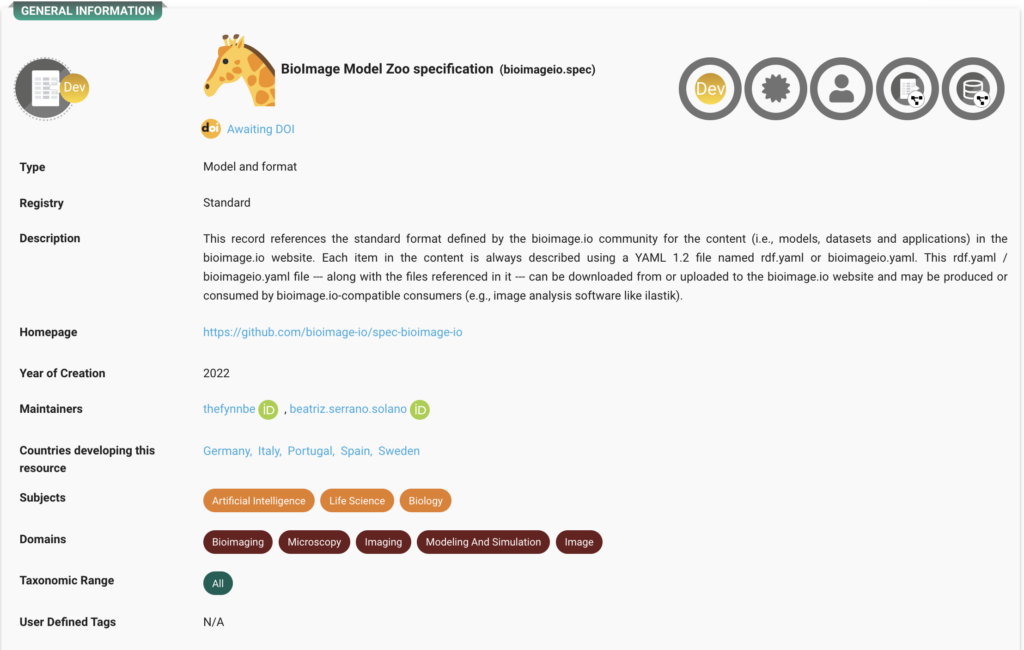
Guidelines for AI-Ready Datasets (MIFA)
Detailed guidance on metadata, formats, and accessibility to make datasets AI-ready and compliant with FAIR standards. Explore the record here.
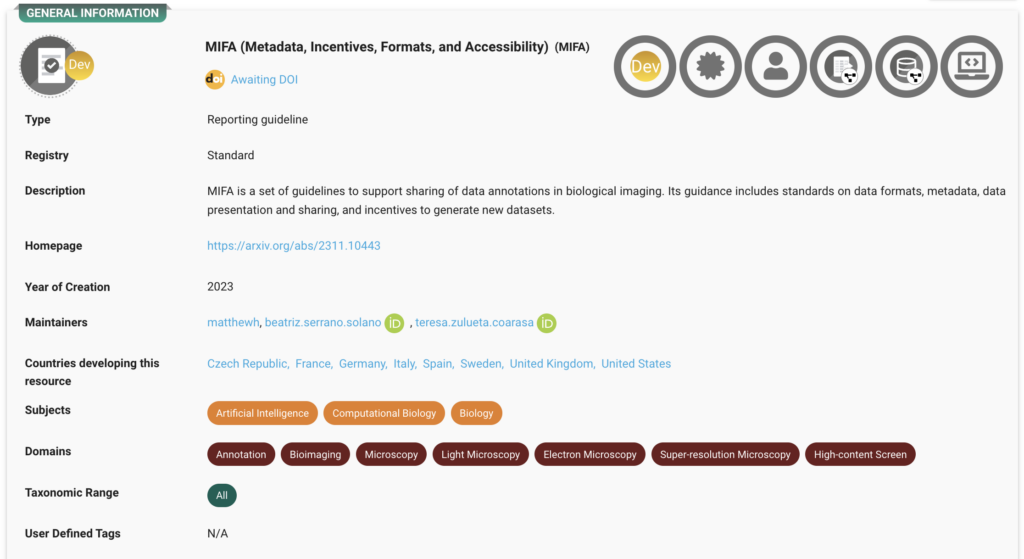
BioImage.IO Repository
The BioImage Model Zoo is community-driven repository of pre-trained AI models. Explore the record here.
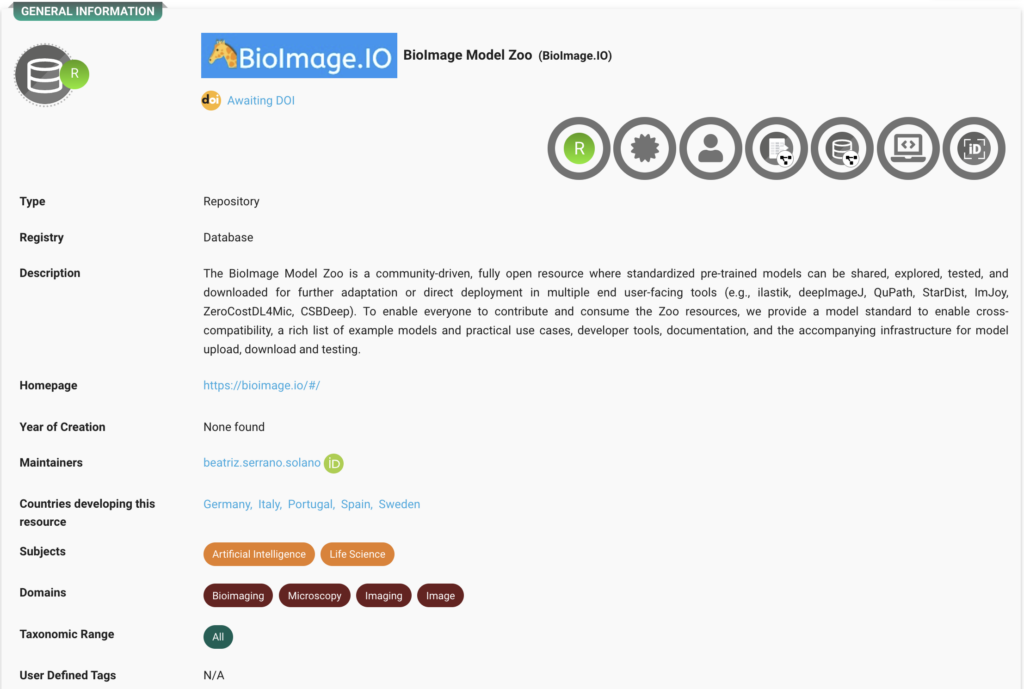
AI4Life Collection
All these records are collected under the AI4Life umbrella record. https://fairsharing.org/collection/AI4Life
For more details on our FAIRsharing records and how they can benefit your research, take a look at our recent deliverable:
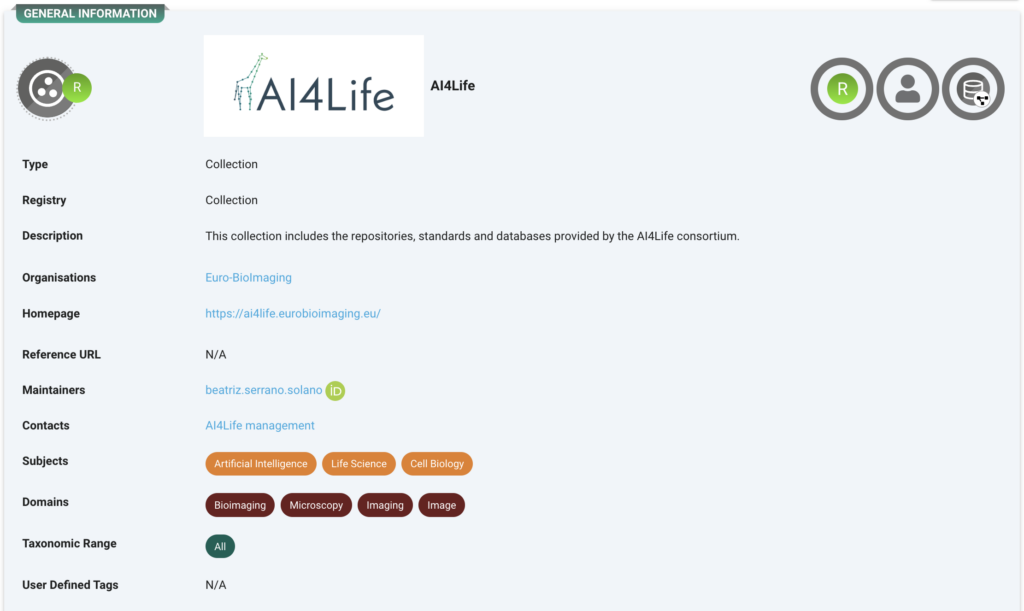